
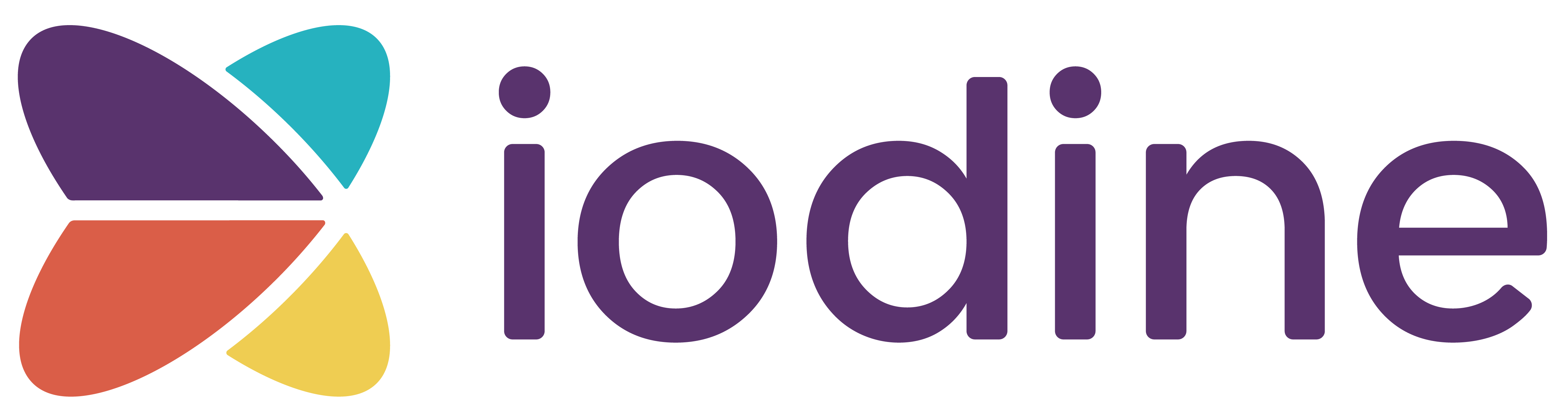
Prioritization and the CDI specialist worklist
By Fran Jurcak, MSN, RN, CCDS, Vice President of Clinical Innovation, Iodine Software
Capturing accurate and complete documentation that appropriately represents the conditions being treated during a patient’s inpatient admission requires a dedicated staff of Clinical Documentation Improvement Specialists (CDIS) with clinical and coding knowledge and a process that allows for regular, detailed review of records. With new clinical evidence and documentation added every day, a daily review of the charts would be necessary to ensure completeness of the medical record. To accomplish this goal, most CDI programs would need to double if not triple their current staff. Unfortunately, most hospitals do not have the resources to implement and train a staff this large let alone the budget to do so.
To maximize the staff’s ability to focus their attention on the records with documentation improvement opportunity, most CDI programs have implemented creative methods to identify which records to review each day. Many programs attempt to prioritize records for review by leveraging clinical experience to take a best guess at which records are more likely to have a documentation integrity concern. This may entail reliance upon the chief complaint, length of stay (LOS), a focus on a particular type of nursing unit or service line, or identification of specific providers who have a history of poor documentation habits. While these strategies can help a CDI department feel like they’re taking some control over a monumental task, none of them specifically surface documentation problems. The unfortunate result is countless complete and accurate charts still being non-productively reviewed while hundreds of records with documentation integrity problems continue to walk out the door without review or correction.
Use of technology to assist CDI staff by creating work-lists may help solve this problem, but prioritizing the actual problems CDI is trying to solve is key. Natural Language Processing (NLP), although an effective solution for tasks which consider only narrative documentation, fails to identify conditions being monitored and treated but which were never (or only non-specifically) documented. Similarly, computer assisted coding (CAC) technologies that utilize NLP to identify working DRGs and create work-lists based upon possible DRG opportunity do not directly address the central CDI problem of finding and correcting discrepancies between evidence and documentation.
An ACDIS survey conducted in July 2017 reported that 54% of responders manually set a date for future review, 48% use the working DRG to identify cases lacking a CC or MCC, and 25% use the working DRG to identify records that have a longer than expected LOS. Although this narrows the focus of potential opportunity, it requires an initial review on every record to determine the working DRG. It also requires a complete review of each record in this smaller group to identify those records with documentation clarification opportunities which may not result in a query opportunity. Prioritizing records in this manner has not been reported to significantly impact query rates and still allows for missed opportunity within the records not reviewed.
An approach that directly identifies likely discrepancies between evidence and documentation would more effectively solve the problem CDI is trying to address. Doing so, though, requires a focus on the discrete clinical data to determine likely conditions, and a consideration of the narrative documentation only within that context rather than as a primary source of diagnostic information. While rules-based approaches have been attempted in this space, they suffer from an unfortunate false-positive/negative dilemma depending on how rules are set. Advanced artificial intelligence provides an escape from this predicament.
Machine Learning (ML) applied to an enormous clinical database can provide an evidence-driven means to predict conditions and their likelihood (probability), providing an escape from the false-positive/negative problem. Applying real time hospital data to these ML models allows for accurate recognition of likely medical conditions for each patient and identifies gaps between actual evidence and documentation to create a list of prioritized patient records for CDI staff review. Following this approach, CDI can focus on the biggest documentation concerns in the organization at any given time.
While the options for ML solutions are limited, the rewards can be overwhelmingly positive. CDI staff are freed from spending the bulk of their time ruling charts out and can focus their attention on the patient records truly requiring their review and intervention. Typical outcomes include significant increases in query numbers without reduction in physician response which results in accurate financial return and reporting of quality benchmarks. A focus on clear and consistent documentation of all conditions being monitored and treated rather than the final DRG supports accurate reporting and decreases risk of denials.